Ruomu Tan, Benedikt Schmidt, Benjamin Kloepper, Arzam Kotriwala, Pablo Rodriguez ABB Process Automation, Corporate Research Ladenburg, Germany, ruomu.tan@de.abb.com, benedikt.schmidt@de.abb.com, benjamin.kloepper@de.abb.com, arzam.kotriwala@de.abb.com, pablo.rodriguez@de.abb.com; Divya Sheel, Chandrika KR ABB Process Automation, Corporate Research Bangalore, India, divya.sheel@in.abb.com, chandrika.k-r@in.abb.com; Anne Lene Rømuld OKEA Kristiansund, Norway Hadil Abukwaik Former ABB employee
Control room operator actions have a significant and direct impact on uptime, product quality and production output as well as safety; basically on all aspects of industrial plant performance →01. To ensure consistent and efficient operation, operators can now draw on the vast amount of available relevant industrial data.
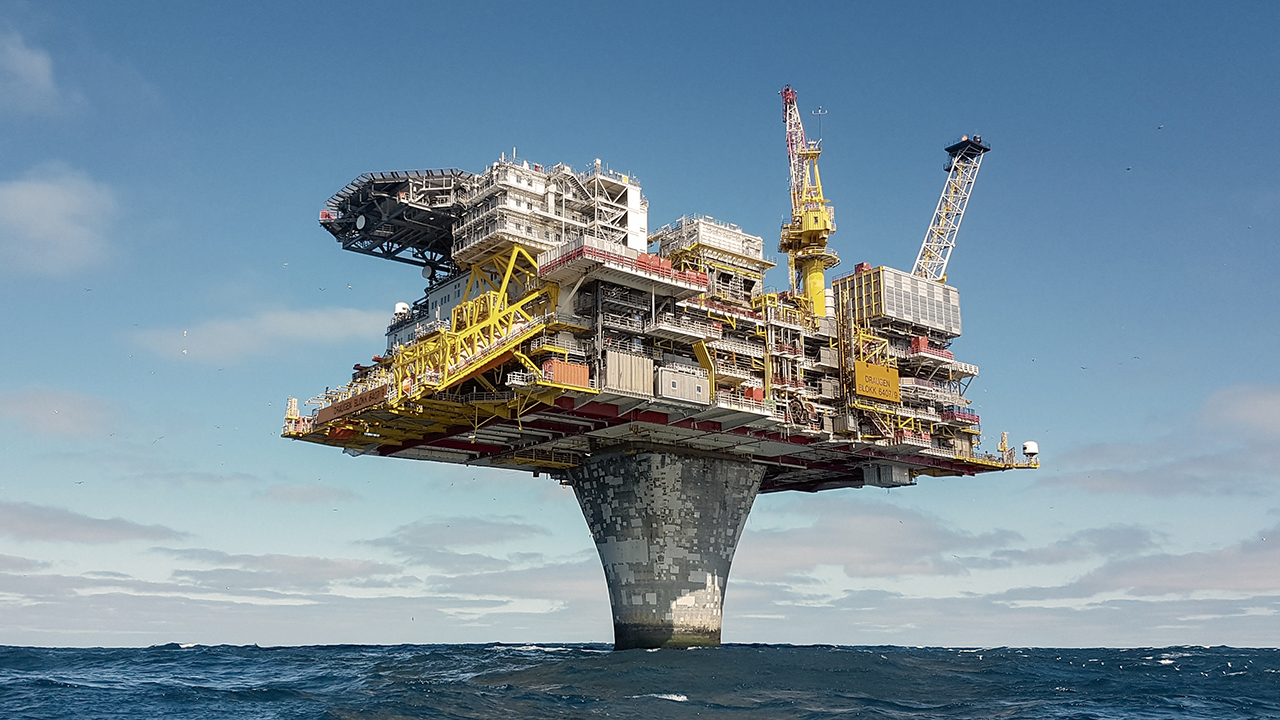
By combining data with deep learning models, profound and transformative opportunities are possible. Recognizing these possibilities, ABB has taken the initiative to develop analytical tools to get the most out of this data.
The Augmented Operator project was initiated in 2020, thanks to a long term association between the oil and gas company, OKEA, and ABB, with the aim of supporting plant operators to achieve operational excellence. ABB developed comprehensive and easy-to-use decision-support tools using deep learning and transformer models, process mining, graph search and causal analysis methods. These tools access and analyze existing data sources such as historical process, alarm and event data, audit trails, engineering documents, standards, and safety procedure. In this way, ABB’s Augmented Operator will help operators to grapple with and resolve abnormal plant situations. Successfully field-tested on data accessed from Norway’s OKEA oil platform, Draugen, ABB’s Augmented Operator meets the strenuous requirements of real-world industrial settings.
The Augmented Operator workflow
Using artificial intelligence (AI) capabilities, ABB’s Augmented Operator workflow was designed to answer five crucial questions every plant operator faces →02:
• Is anything wrong?
• Why does it go wrong?
• What can be done?
• Will it work?
• Has it happened before?

Is anything wrong?
To determine if anything is amiss, the Augmented Operator uses deep learning models, with Long Short-term Memory (LSTM)/Recurrent Neural Networks (RNN) and Convolutional Neural Network (CNN) and Autoencoders, trained on process data to support operators in monitoring. These models are capable of detecting deviation – signatures of potential undesired behavior in the process – early enough to allow operators enough time to intervene and prevent a system shutdown. In addition to timing, the ease of access of such information is essential. Hence, the deep learning models are created in a way that enable customer self-service; the input signals of the models are derived from operator screens and process topology. The Augmented Operator can also highlight the tags that are responsible for the current anomaly so that users can take specific actions accordingly.
The detection of anomalies in process data is the entry point of the Augmented Operator workflow →02. When early signatures appear, they will be detected as an anomaly by the models. Relevant information about the anomaly will also be provided to the operator, eg, what the operator can do to prevent the anomaly from developing into more severe problems or to mitigate the impact on the process. The information will also be communicated to other functions of the Augmented Operator.

In order to circumvent interoperability issues, the Anomaly Detection functionality was designed to be compatible with ABB’s digital tools →03 and has been onboarded onto ABB’s Genix™ Model Fabric. Here, it can receive time series data, calculate the contribution of the anomaly indicator and the process variables, thereby providing information about the process performance in real-time →03.
Why does it go wrong?
Another advantageous feature is the root-cause analysis support function →03. Here, this function, receives the abnormal tags from the anomaly detection model and detects the process signals and actuators that are potentially related. It analyzes the existing engineering artifacts, including various diagrams and the process topology model among others, and the operation information. In addition, live- and historical event and alarm data and variables are used to derive the context for a set of triggered alarms. The produced result is a novel presentation of alarms, plus detected anomalies, with contextual enrichment, including the dependency relationships based on the topology model of the process, or the plant, and the chronological ordering of all related alarms →04. Such features help to reduce the operators’ manual challenge of actually finding the necessary information and mentally capturing the information during his/her analysis [1-3].
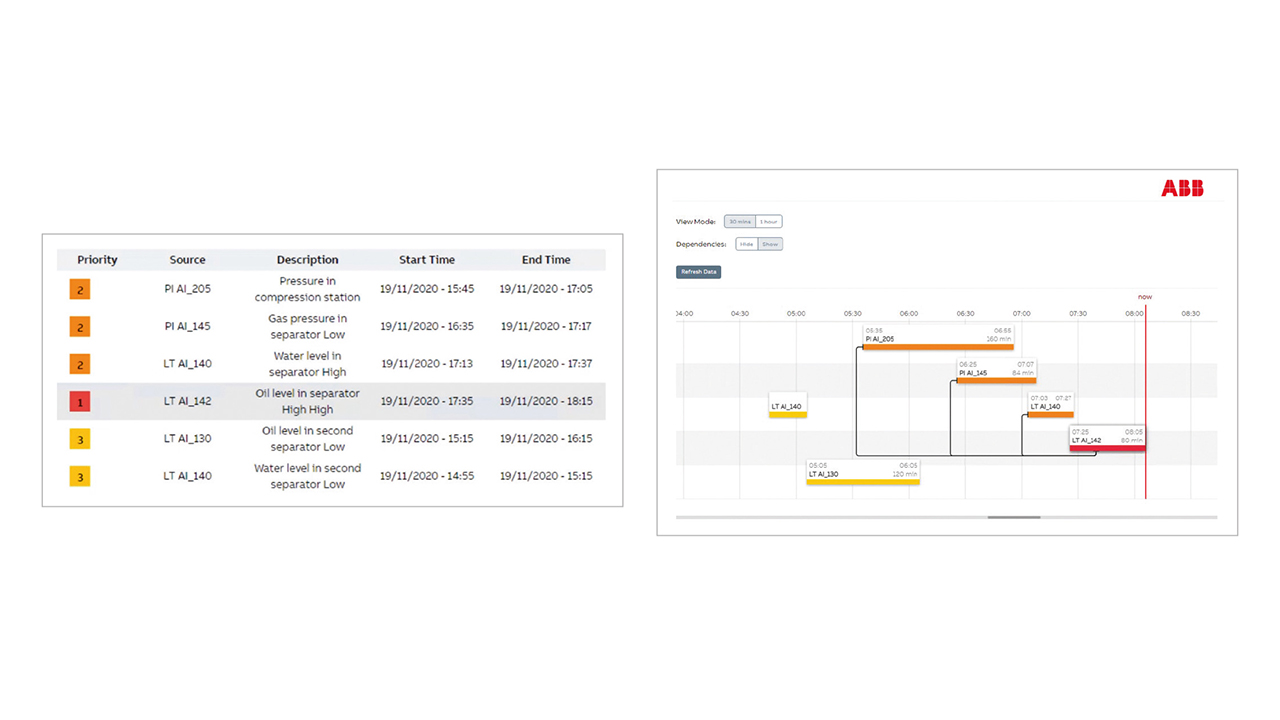
ABB’s solution relies on Transfer-entropy to statistically confirm the presumed causal relationships derived from the plant topology model. The system highlights those actuators identified as the process variables, within the process model, that have the most contributory influence on the undesired event. By highlighting these actuators, the operator can more easily decide how to amend an undesired situation. By ascertaining the causal relationships, the operator can troubleshoot and resolve problems with ease.
What can be done?
Not only must operators unlock potential problems that arise, they must discern what they can do to rectify or modulate difficulties →05. Because the Augmented Operator learns from previous historical operator actions and can generalize this knowledge into workflow documents, operators can now decide how best to deal with any problematic situation.

While expert operators probably know which actions are required under most circumstances, except perhaps for unusual and difficult situations, novices might even find routine situations taxing. The Augmented Operator supports all operators, experts and novices alike, with blueprints for process amelioration from the workflow documents.
The plant historian records an audit trail of the operator’s interactions within the plant. This log of interventions is used to learn how operators have previously interacted with the plant. The log includes hints as to the opening- and closing of valves; overrides of systems and set point changes. By analyzing the temporal flow of the historical actions, reaction times and waiting times between actions can also be extracted. Using this information, workflow mining techniques allow blueprint processes for different plant situations to be extracted →06. Common situations that can be analyzed via this process are alarm response activities, and startup- or shutdown of components – fostering operator decision-making →06.

Will it work?
Just providing operators with possible steps that enable blueprints for various plant situations is not enough to ensure smooth operations under anomalous situations; the operators must be convinced that their actions will work. The Augmented Operator provides this certainty.
Once a possible course of action is identified from historical operator actions, operators can test this procedure for any undesired side effects using a “what-if” tool. This highly desirable feature allows operators to test actions such as set point changes or actuator positions, before implementing them in the real-world system.
It must be noted that there are benefits and challenges associated with such a tool. Although, process simulators appear to be the perfectly matched tool for such functionality, complexity can be an issue for some industries. For processes of moderate complexity, as typified by the chemical, oil and gas industries, such simulators achieve only a speed-up factor of 5- to 10-fold compared to the actual real process. If a process takes around one hour to settle after an action is implemented, then the operators must wait between 6 and 12 minutes to initiate a response. Such a response time duration is simply too long to allow the operator to test several alternative action courses in an interactive and iterative fashion – a potential disadvantage that could, in certain cases, increase risk.
To obviate the short comings of first-principle-based process simulators, machine learning-based surrogate models have been developed for the Augmented Operator workflow. The key here is to capture the process transitions correctly, including aspects like overshot/undershot with regard to alarm limits or the time-lapses that occur until steady-state is reached following the implementation of an operator action.
Testing the “what if” model approach
The Augmented Operator system approach has now been applied to the Norwegian OKEA Draugen Oil Platform →02 using its 800xA Simulator system and the availability of rich historical data gathered from the collaborative operation center. The previously described what-if model was tested using specifications given by the Draugen operators. The objective was to capture operator changes made to the rotation speed (RPM) of one of the main pumps in Draugen’s water reinjection systems; and to predict, ten minutes ahead, the impact of these RPM changes on the related trip signals encountered in the downward flow to the well. For this purpose, a Deep Neural Network (DNN) with a LSTM architecture was trained to predict the platforms’ response to changes in RPM →07. Such Deep LSTM Neural Network architectures have proven suitable for multi-dimensional time series forecasting using, eg, Tensor Flow, because they can keep a context of memory to attack temporal and spatial problems. The successful run of the model in a real-world environment – an oil platform – lends credence to the efficacy of this tool in moderately complex process industrial environments.

Has it happened before?
The Knowledge Extractor of the Augmented Operator provides an alternative way to gain further valuable insights into possible plant incidents and to perform scenario studies by analyzing similar past situations →07.
Consider an incident in which an operator observes that water and oil level set points in a scrubber are too close, thereby leading to strong interactions. The operator might wish to know if this happened previously. Currently, (s) he will investigate shift logs and previous trips to compare similar events; this is a manual and cumbersome process. The Knowledge Extractor component enables operators to snip a part of the trend that is of interest and to search for similar set point changes in the process data that occurred previously →08.
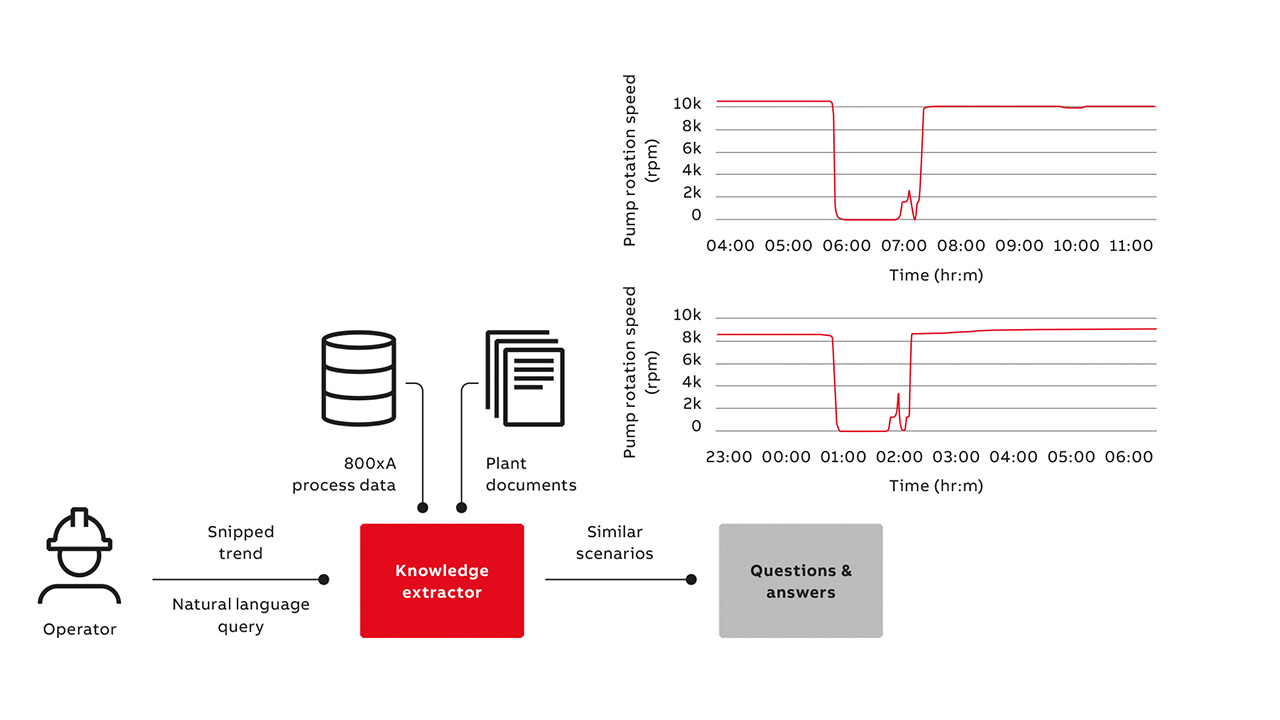
The Knowledge Extractor also allows fast access to written documents eg, incident reports, routinely received from service engineers, analysts, and other engineering firms, by posing natural language questions such as “What should be done if the oil and water level set point are too close in the scrubber?”.
Building and testing the Knowledge Extractor
The Knowledge Extractor uses time-series-based pattern mining techniques to perform similarity searches to find particular scenarios of interest from the process history data →08. Moreover, deep learning models were used to understand natural language, especially the intent behind words. For this purpose, pre-trained deep Natural Language Processing (NLP) models that are based on the latest Google publications [4,5] were fine-tuned to generate a novel contextual question-answering system. The research team at ABB developed multiple prototypes in order to answer the questions posed to the system.
This prototype was tested on the trip data from the real-world Draugen oil platform where it was applied to specific scenarios. For example, whenever gas pressure increases over a specific threshold, the pump trips, and the level of the feed-water rises in the degassing tank. Relevant trip data was generated whenever an operator restarted a pump multiple times after a trip occurrence. By using the Knowledge Extractor tool, similar trip scenarios could be identified from the historical process data →08. Moreover, the ability to pose natural language questions and access tuning service reports from previous years enabled operators to determine that low suction pressure caused the multiple pump trips.
By designing Augmented Operator to address five crucial questions, ABB has created a tool that will not only be able to access vast amounts of data, identify and analyze anomalies, past and present, but also provide solutions for operators that will work. Aptly demonstrated and validated on a rich data set supplied by OKEA, ABB’s innovative solution has proven its viability in real-world industrial environments.
References
[1] D. Reising, etal., “Human performance models for response to alarm notifications in the process industries: An industrial case study,” in Proceedings of the Human Factors and Ergonomics Society Annual Meeting, Los Angeles, CA, 2004.
[2] A. Kabir, et al., “Similarity analysis of industrial alarm flood data,” IEEE Transactions on Automation Science and Engineering, vol. 10, no. 2, pp. 452 – 457, 2013.
[3] Y. Niwa and E. Hollnagel, “Enhancing operator control by adaptive alarm presentation,” International Journal of Cognitive Ergonomics, vol. 5, no. 3, pp. 367 – 384, 2001.
[4] Raffel, Colin, et al. “Exploring the limits of transfer learning with a unified text-to-text transformer.” in JMLR, Vol 21, issue 140, 2020, pp. 1-67.
[5] Kwiatkowski, Tom, et al. “Natural questions: a benchmark for question answering research.” Transactions of the Association for Computational Linguistics, Vol. 7, 2019, pp. 453-466.