ABB developed a four-step co-innovation approach for analytics and artificial intelligence projects. Leveraging engineering domain knowledge and data science expertise, the approach allows ABB, partners and customers to create advanced analytics and artificial intelligence solutions together.
Benjamin Kloepper, Martin W. Hoffmann ABB Corporate Research Ladenburg, Germany, benjamin.kloepper@de.abb.com, martin.w.hoffmann@de.abb.com; James Ottewill ABB Corporate Research Krakow, Poland; james.ottewill@pl.abb.com
Advanced analytics and artificial intelligence (AI) applications are gaining traction in industrial automation, thereby enabling higher levels of autonomy [1-2]. Nevertheless, AI is complicated, and combining it with automation does not in and of itself generate higher value to a project: Additional value requires focus, high-level skills and enough reliable data. When advanced analytics and AI are applied to relevant well-defined opportunities, considerable additional value can be unlocked as an integral part of an end-to-end solution. The right set of data science expertise, clear domain understanding and engineering knowledge working in concert can ensure this value. Collaborative research and development, where knowledge and expertise are shared and leveraged can underpin this process. With the right experts and experience available, ABB has developed a standardized co-innovation approach to orchestrate this essential collaboration.
Loosely based on the CRISP-DM approach [3], ABB’s new four-step systematic approach has been adapted to run co-innovation projects in advanced analytics, machine learning and AI with partners and customers. While this approach is described as a four-step approach, it is, in practice, an iterative process as knowledge and understanding generated during the collaboration leads to further ideation. ABB’s experts have, over the past few years, implemented this process with customers across a gamut of industries, eg, chemicals, automotive and utilities, to focus, use and generate quality data to deepen value of advanced analytics and AI projects [4-5].
Four steps to value: co-innovation
The co-innovation scheme defines processes and objectives in well-structured steps so that automation providers and customers can know where they are and where they want to be at any given time during a project →01.

• Co-innovation step 1: identification and value proposition articulation
• Co-innovation step 2: data inspection and collection
• Co-innovation step 3: AI and analytics modelling
• Co-innovation step 4: deployment
Step 1: Beginning with the identification phase, face-to-face workshops with customers and ABB stakeholders identify “pain points”, or problems and issues, relevant stakeholders and then develop a value proposition – the promise of a value or perceived value to be communicated, delivered and acknowledged. The industrial AI problem is thus formulated.
Step 2: Access to the right data with the right quality is paramount to the success of advanced analytics and AI development projects. Data inspection and collection ensures these needs are met.
First, domain and data scientists identify the data needed to address the industrial AI problem through day-long workshops or interviews, thereby facilitating knowledge-sharing.
Second, the suitability of data already available is assessed. Missing data is identified. The experts also consider how fusing heterogeneous data from a variety of sources (eg, signal data, alarm and event data, business data) might also support the realization of the value proposition.
If data quality or quantity is insufficient, a data collection campaign can be planned, additional sensors installed, or data from a non-obvious source can be substituted for missing data [6].
Step 3: ABB’s AI modelling experts begin this phase by exploring the data and preparing it for modelling. Remaining data quality issues are detected and treated [7], correlations are identified, features are designed and hypotheses are generated. Lessons learned from this phase are used to fine-tune the industrial AI problem.
The ‘Train-Validate-Tune Test Cycle’ is initiated next. Here, the data scientist designs and trains data-driven models, corroborates the model on a validation data set (or in cross-validation) and refines the model hyper parameters or re-engineers features as needed. These approaches vary from purely data driven, such as neural networks, to models based predominantly on the laws of physics, and include everything in between. Hybrid approaches are developed to leverage the strengths and mitigate the weaknesses of each individual model. By combining domain and data science expertise, the design of the model is guided: from properly defining model inputs, outputs and structure to selecting the appropriate modeling approach and defining a cost function that accurately quantifies the performance of the model.
Upon validation, the model is tested on a new data set, one that the algorithms have not been trained on. Furthermore, model interpretation tools are used to investigate the reasoning within black box models like random forests or artificial neural networks.
Mock-up user interfaces, based on real data and predictions, are created early on, thereby boosting modeling and workflow evaluation. And, by continuously sharing results and knowledge with stakeholders and customers during this phase, ABB receives crucial feedback to improve the model.
Step 4: During the deployment phase, the data pipelines and machine learning workflows from the AI modeling phase are operationalized. An in-place system is required for retraining the machine learning models (eg, retraining on request, scheduled or based on some event). A software system is used for running the scoring of the machine learning model and making the output available to the user.
Together with the customer, ABB decides how to deploy the AI solution, eg, as a web dashboard, integrated in existing software on-site or perhaps as a virtual assistant.
Use case: performance monitoring in a solar power plant
ABB’s four-step research and development approach has been successfully deployed to create an advanced analytics solution for industrial automation in utility and process industries, among others.
In one case, ABB’s co-innovation approach helped domain and data science experts from the power grids and electrification businesses and research and development teams in Poland, China, Sweden, Switzerland and Germany deliver an innovative customer advanced analytics solution to monitor the performance of photovoltaic plants. The four-step solution for a solar plant is given below.
Step 1: Condition monitoring systems can increase uptime and yield, and ultimately decrease the life-cycle costs of a solar production plant. However, the distributed and modular nature of solar plants presents challenges. The remoteness of such plants and their typical unmanned operational set-up compounds these challenges. As a result, operators require very accurate and cost-effective monitoring systems that relay the current performance and health of a plant and pinpoint the root cause of any potential problem.
Step 2: The costs associated with installing, configuring and maintaining an independent condition monitoring system, with tailored high-end sensors, cabling and communication requirements, would quickly and adversely impact the value derived from any monitoring system. However, as a provider of advanced industrial digital technologies, ABB was also acutely aware that solar plants already use significant acquisition and storage systems, eg, SCADA systems, remote terminal units, inverters and maintenance management systems. Drawing on domain knowledge of photovoltaics, power electronics, automation and condition monitoring applications ABB evaluated the usefulness of this data relative to the value proposition to properly formulate the analytics task.
Step 3: Seizing on comprehensive domain knowledge and strong analytics fundamentals, ABB scientists designed and implemented advanced methods to solve the analytics task: inputs, outputs and cost functions of data-driven models for components within a plant were properly formulated. The resulting system is able to extract meaningful actionable insights from the data →02, eg, degradation rates, fault diagnosis and root cause analysis.

Step 4: A holistic solution was developed by contemplating all analytics steps from data ingestion, through data cleaning to model preparation and deployment. By considering the user experience throughout the process, ABB could increase comprehension and transparency. Currently the development is included as an application aspect of e-mesh™ Analytics Suite, and will be an application that runs on ABB Ability™ e-mesh™ Monitor digital solution →03, which builds on the cloud-based digital platform that aggregates data from distributed energy assets. The novel solution is easy-to-deploy and scalable, while providing a single location to obtain business insights from multiple assets.
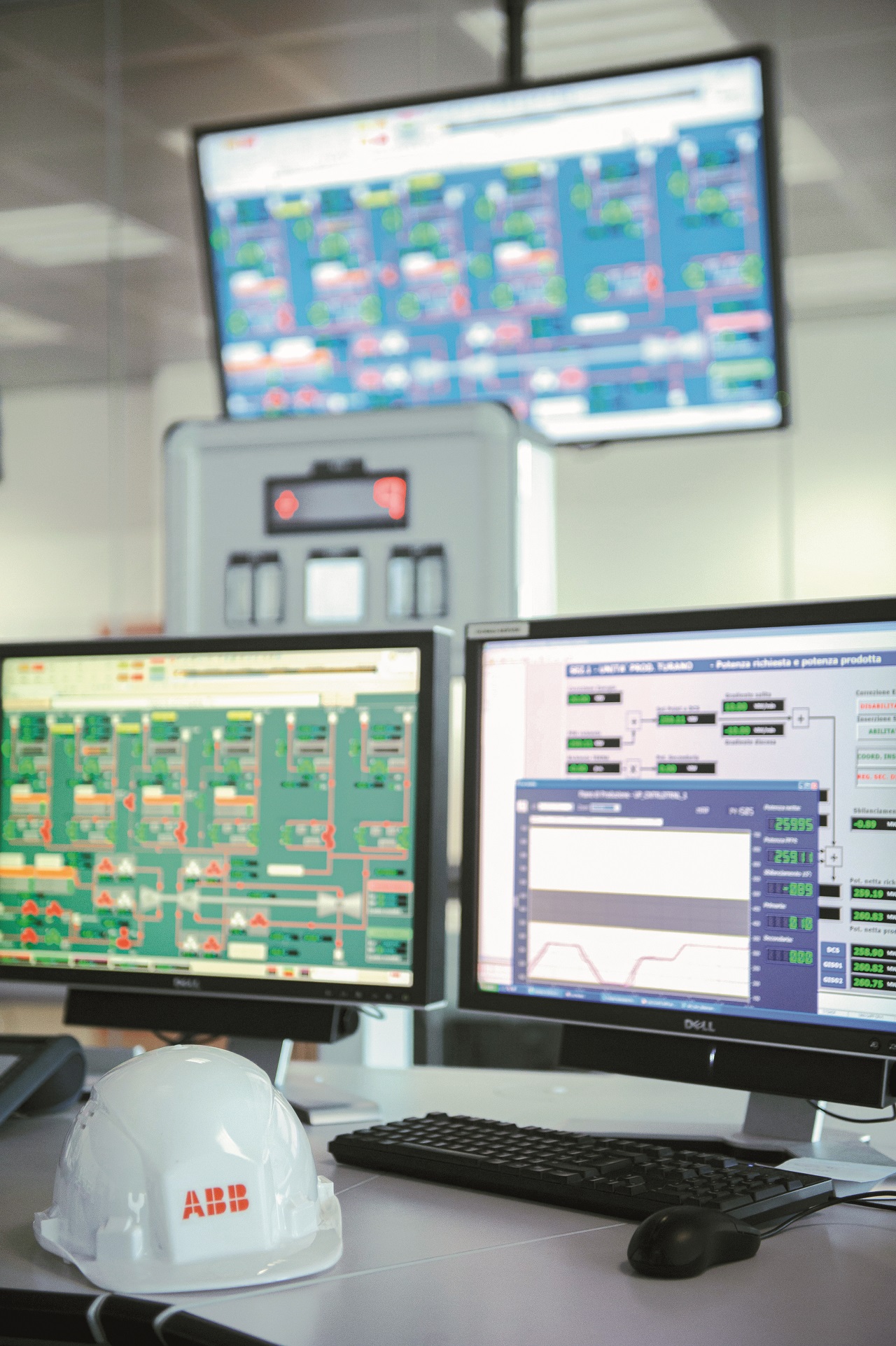
Use case: predictive maintenance for standard rotating equipment
ABB also applied the co-innovation method to develop a solution for performing predictive maintenance of rotating equipment in a process plant →04 [8-9].

In this case, customer stakeholders, including plant managers, operators and reliability engineers collaborated with ABB data scientists, rotating equipment asset experts and design-thinking practitioners to create a value proposition to enable predictive maintenance for rotating equipment. There are typically numerous low voltage motors and pumps present in such a plant. Equipment breakdown for this type of equipment and consequent unscheduled maintenance activities are much costlier than a planned maintenance activity. Due to the number of such devices, it is not feasible to manually record data and analyze the health state of each device so, they are usually run to failure, thereby resulting in high overall asset replacement costs.
Step 1: The final value proposition was formulated: ‘Safeguard operations against unscheduled breakdown of standard pumps within the next two weeks’. This was translated into an analytics task: Predict if a pump will fail within the next two weeks and, if yes, why will it fail.
Step 2: The data inspection yielded the result that the data, which was already collected, was insufficient for the analytics purpose. Condition monitoring systems were only deployed to large, higher-value pumps. And yet, lower-cost devices can also significantly impact maintenance costs. These devices were not monitored to the same extent. The customer identified, with the support of ABB, a pilot plant to install ABB’s wireless sensing technology to generate the required data. ABB set up a suitable data collection infrastructure so that ABB’s data scientists could also access the data.
Step 3: ABB’s data scientists and asset experts analyzed the incoming data and could identify indications of potential faults →05. Cases in which symptoms of faults were identified were immediately shared with the customer who was able to investigate and confirm the detected problems. With data samples from healthy systems and confirmed failure cases, ABB’s data scientists were able to train a deep learning model that satisfactorily predicts if a pump would fail within the next two weeks.

Step 4: The research work on predictive maintenance for standard pumps will become part of the ABB asset performance portfolio: a value-added service offering in which ABB’s asset experts and customer maintenance managers monitor equipment that is supported by ABB’s artificial intelligence algorithms [9].
Be part of the co-innovation process
Relying on their novel 4-step framework to support collaborative research and development, ABB could efficiently develop tailored industrial AI solutions for multiple clients. Data scientists and domain experts, customers and stakeholders working together and sharing knowledge adds substantial value to this endeavor. ABB invites its customers and partners to collaborate with their data scientists and domain experts to experience this illuminating process for themselves and to adapt it to their specific project needs.
References
[1] T. Gamer and A. Isaksson, “Autonomous systems,” ABB Review 04/2018 pp. 8-11.
[2] T. Gamer, et al., “The Autonomous Industrial Plant – Future of Process Engineering, Operations and Maintenance,” in 12th International Conference on Dynamics and Control of Process Systems (DYCOPS), vol 52-1, 2019, 435-460.
[3] R. Wirth and J. Hipp, “CRISP-DM: Towards a standard process model for data mining,” 4th International Conference on the Practical Applications of Knowledge Discovery and Data Mining, 2000, pp. 1-11.
[4] B. Schmidt, et al., “Industrial Virtual Assistants: Challenges and Opportunities,” ACM International Joint Conference and International Symposium on Pervasive and Ubiquitous Computing and Wearable Computers, Singapore, 2018.
[5] M. Atzmueller, et al. “Big data analytics for proactive industrial decision support,” atp magazin vol 58-09, 2016, pp 62-74.
[6] J. Ottewill, et al., “What currents tell us about vibrations.” ABB Review 01/2018, pp.72-79.
[7] R. Gitzel, et al., “Data Quality in Time Series Data: An Experience Report.” 18th IEEE Conference on Business Informatics (CBI), 2016.
[8] I. Amihai, et al. “An Industrial Case Study Using Vibration Data and Machine Learning to Predict Asset Health.” 20th IEEE Conference on Business Informatics (CBI). 2018.
[9] R. Gitzel, et al., “Transforming condition monitoring of rotating machines,” ABB Review 02/2019, pp. 58-63.