Martin Hollender, Benedikt Schmidt ABB Corporate Research Ladenburg, Germany, martin.hollender@de.abb.com, benedikt.schmidt@de.abb.com; Chaojun Xu ABB Industrial Automation, Energy Industries Mannheim, Germany, Chaojun.xu@de.abb.com; Moncef Chioua Department of Chemical Engineering, Polytechnique Montréal, Montreal, Canada
Big data processing architectures like Hadoop or Spark enable new possibilities to analyze and exploit historical data generated by process plants [1,2]. Predictive maintenance, operation support, soft sensing for monitoring and control, and integration of control and enterprise resource planning (ERP) layers are just some of the envisioned applications of such technologies in the process industry sector.
One area in particular where big data can be exploited to make significant improvements is the batch process industry →01. Accordingly, ABB, in collaboration with experienced end users at a pilot customer, has developed ABB Ability™ BatchInsight, an operator support system able to detect and troubleshoot abnormalities in a batch in real time. This online system helps operators to run plants in a smooth and trouble-free way as emerging process issues can be detected at an early stage and corrective actions taken while the batch is running.
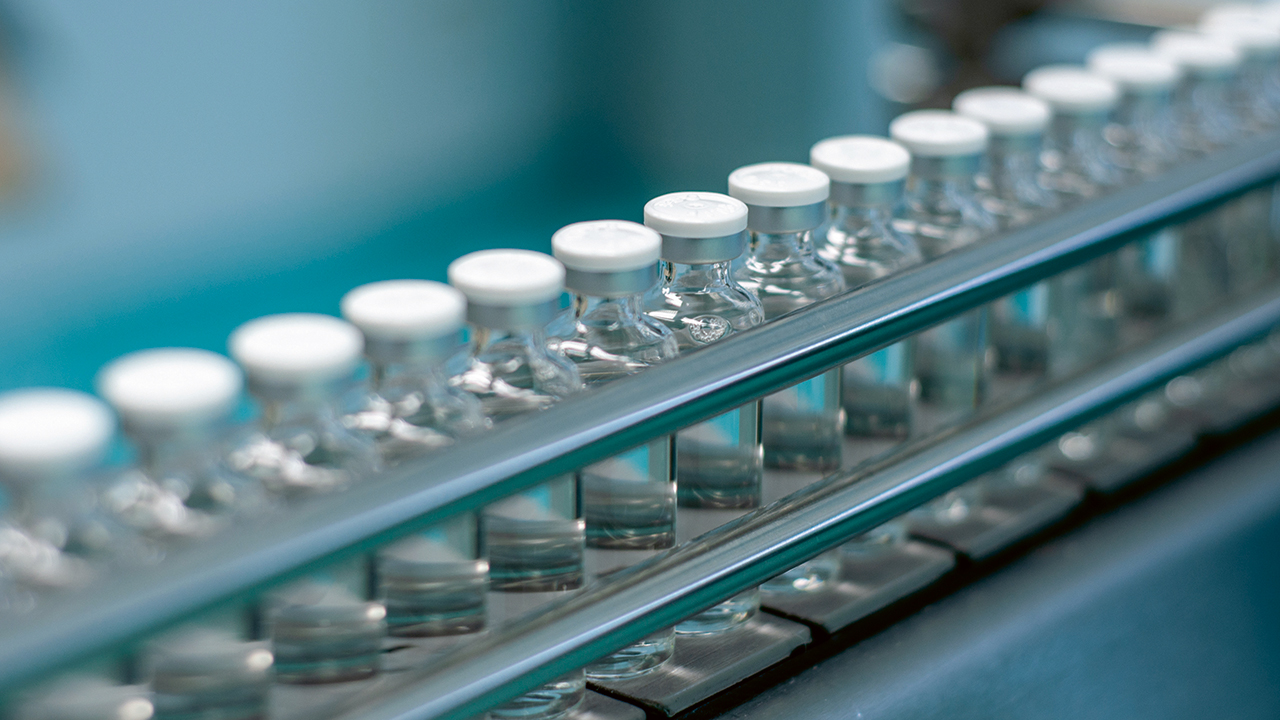
The adopted approach uses historical data to learn the expected behavior of the batch process under nominal conditions and builds a statistical “golden batch” model, which is then used as a reference for the batch currently under production. Deviations from this golden batch model generate a warning to the operator.
Application scenario batch analysis
More than 300 batch runs from a period of over two years were sourced from the pilot plant and used to generate a statistical golden batch model reflecting all the possible good batch behaviors →02.
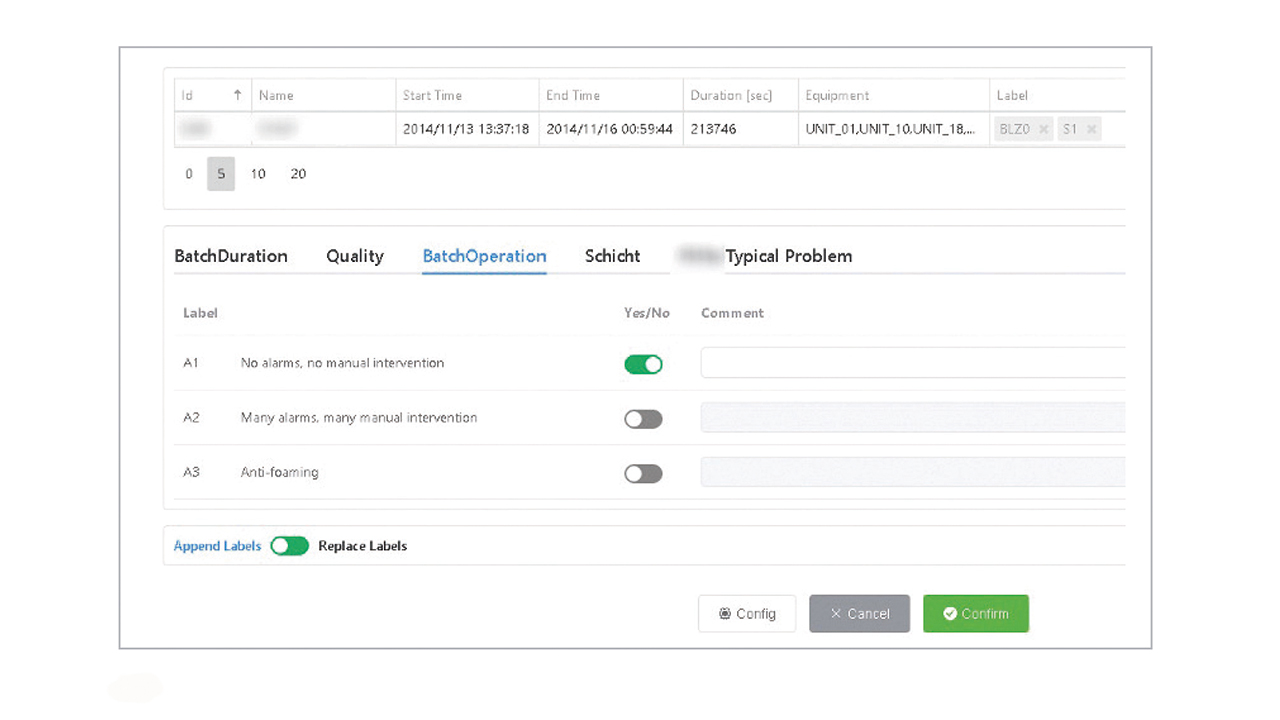
As a first step, these batches were labelled as “good” or “bad” – an exercise that is often not as straightforward as it first seems. For example, a batch might have started to go in the wrong direction, but then the operator intervened and made sure that the batch was produced smoothly – ie, in time and with good quality. From the perspective of the plant, this is a good batch, whereas, from the perspective of machine learning, it is a bad batch, because without the operator intervention a production problem would have occurred. To complicate matters, the operator may also have intervened for a reason that has nothing to do with potential unwanted behavior. During production, it is usually clear to the operators what is going on, but later on it is sometimes much less obvious why the trend curves look the way they do; important operational context can get lost. Modern big data analytical tools depend on such context information. It is crucial to develop a system to record this information and train and motivate operators to use it appropriately.
→02 shows an example of a ABB Ability™ Batch-Insight batch label dialog. It can be easily extended with additional label groups. In this example four groups are shown: BatchDuration, Quality, BatchOperation and Shift (“Schicht”) and individual labels. The currently selected label group is marked blue and the labels it contains are shown below. The batch label dialog can be opened from all places where batches are involved. It is also possible to assign labels automatically via scripts. Later on, when the analyst needs to decide which batches are used to build the model, the labels can be of critical assistance.
The model editor is used to select appropriate historical batch runs for the golden batch model →03. In addition, known good batches and known bad batches can be used for verification to ensure that the model has a sufficient quality level.
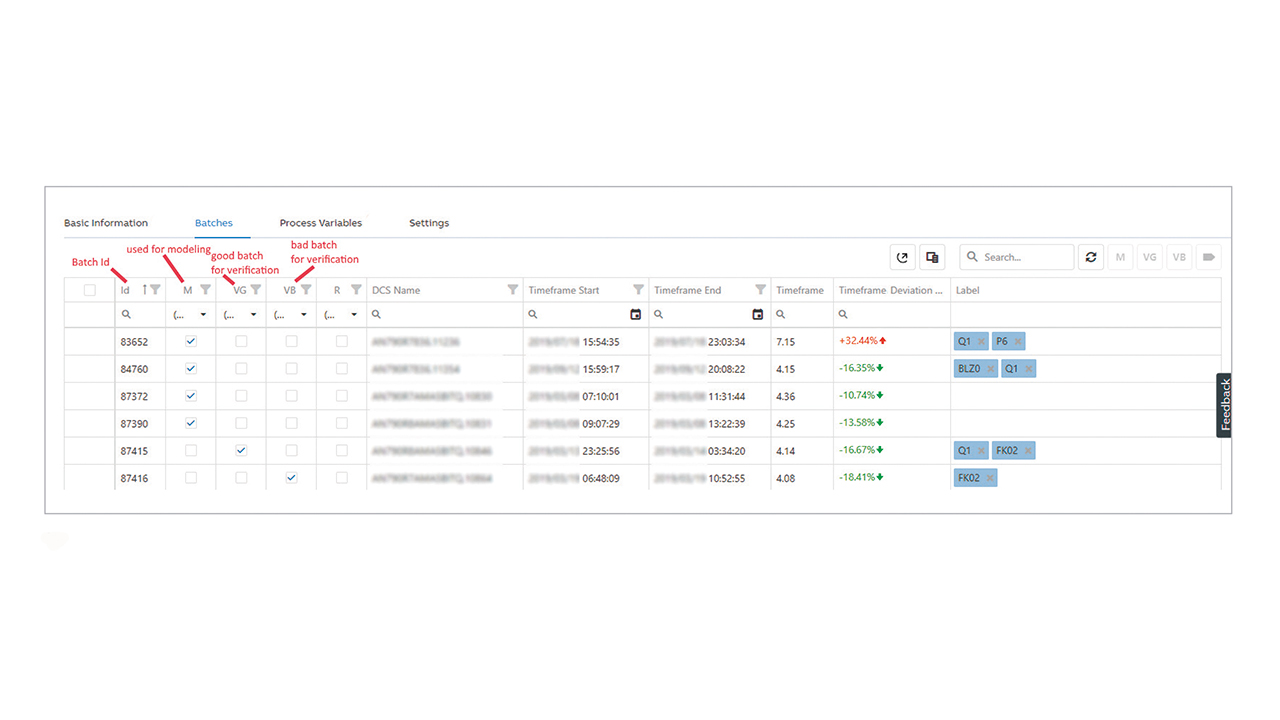
Batch alignment
ABB Ability™ BatchInsight contains a detailed model of a batch, as defined in ISA88, a standard addressing batch process control. This model includes timestamps of the start and end of each batch operation and phase. Batch durations differ from one batch to the next. Dynamic time warping (DTW) – a time alignment technique – is used to stretch or compress the datasets to make them comparable without losing or changing the dynamic characteristic of the data.
Alternatively, a computation of similarity between batches can be used to generate a similarity index that can be compared with a reference value. This approach allows a finer and more efficient analysis: because a group (or “cluster”) of batches might exhibit the same types of abnormalities, the diagnosis could be made only for a subset of these batches. Ideally, such clusters would separate good and bad batches.
Dimensionality reduction
While clustering allows batches that are similar to the reference (nominal/healthy) batch to be separated, more details are required in order to diagnose faulty batches. A multiway principal component analysis (MPCA) [3] is, therefore, used to analyze the reasons for the batch failure. This approach focuses on the process variables that statistically explain the detected batch variability. An online implementation of this technique allows early detection of an abnormal evolution of an ongoing batch.
Estimation of batch quality
One key ambition of a batch operation is to ensure that the product target quality is reached. Current industrial practice is to take samples from the batch during its evolution and send them for laboratory analysis. Based on the analysis results, the process operator adjusts the batch process variables and the batch process duration accordingly.
Partial least squares (PLS) regression is a linear dimensionality reduction method that allows the statistical analysis of highly correlated large multivariate datasets. Batch process quality monitoring relies on a variant of PLS – multiway partial least squares (MPLS).
An MPLS model is a regression model that relates the batch quality to the batch process variables, acting, therefore, as an inferential (soft) sensor able to tackle a crucial issue in the process industry: the lack of reliable online quality measurements. In addition, MPLS is able to predict quality measurements hours before they become available from the lab. In some cases, this can shorten waiting times and lead to better asset utilization.
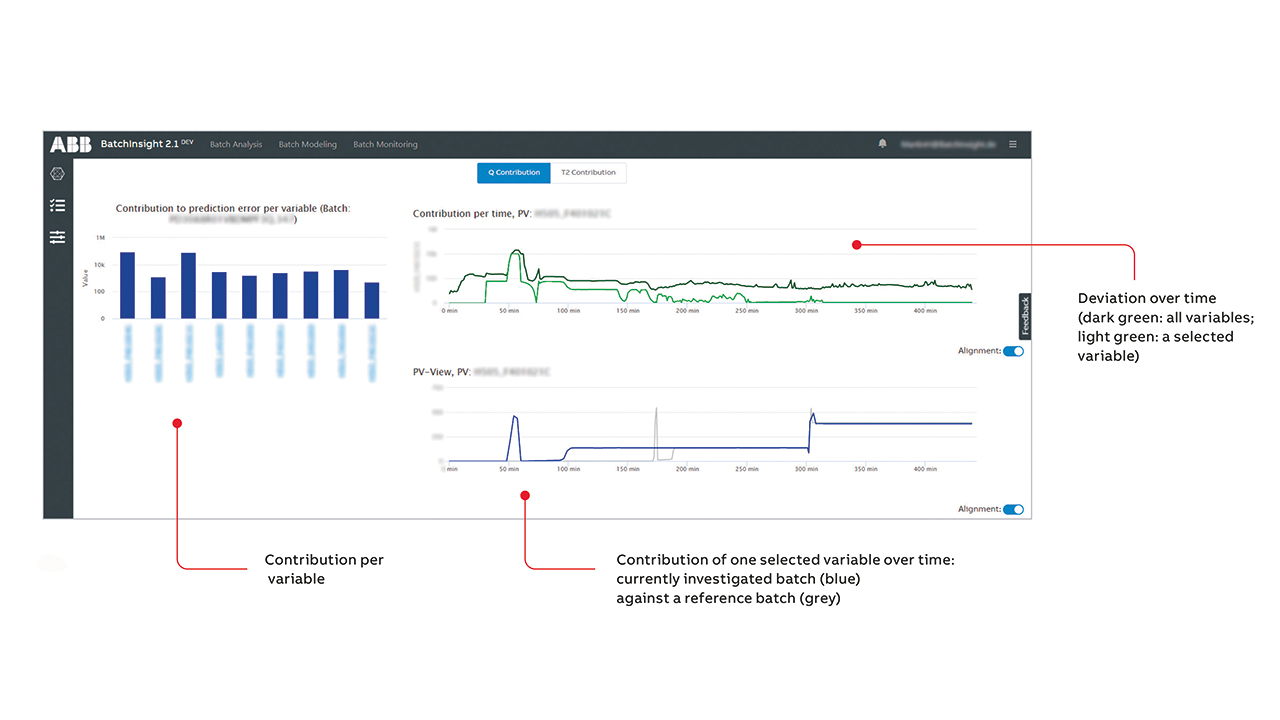
Diagnosing the details
Once ABB Ability™ BatchInsight detects a significant deviation from the golden batch model, the operator’s display shows what is different →04. Compared to a single-variate analysis, multivariate analysis techniques can take correlations between variables into account.
Supporting the expert
Batch processes can be complex, even for domain experts: Good/bad batch decisions must be taken, statistically relevant process variables need to be identified and, in the end, fine-tuning is required. Therefore, ABB Ability™ BatchInsight includes novel techniques that support the preparation of the process analysis, including automatic identification of relevant variables for the different phases of the batch process and an automatic proposal of batches to use for the MPCA and MPLS models. These techniques act as recommenders and free up the expert to investigate core issues using ABB Ability™ BatchInsight’s analytics techniques →05.
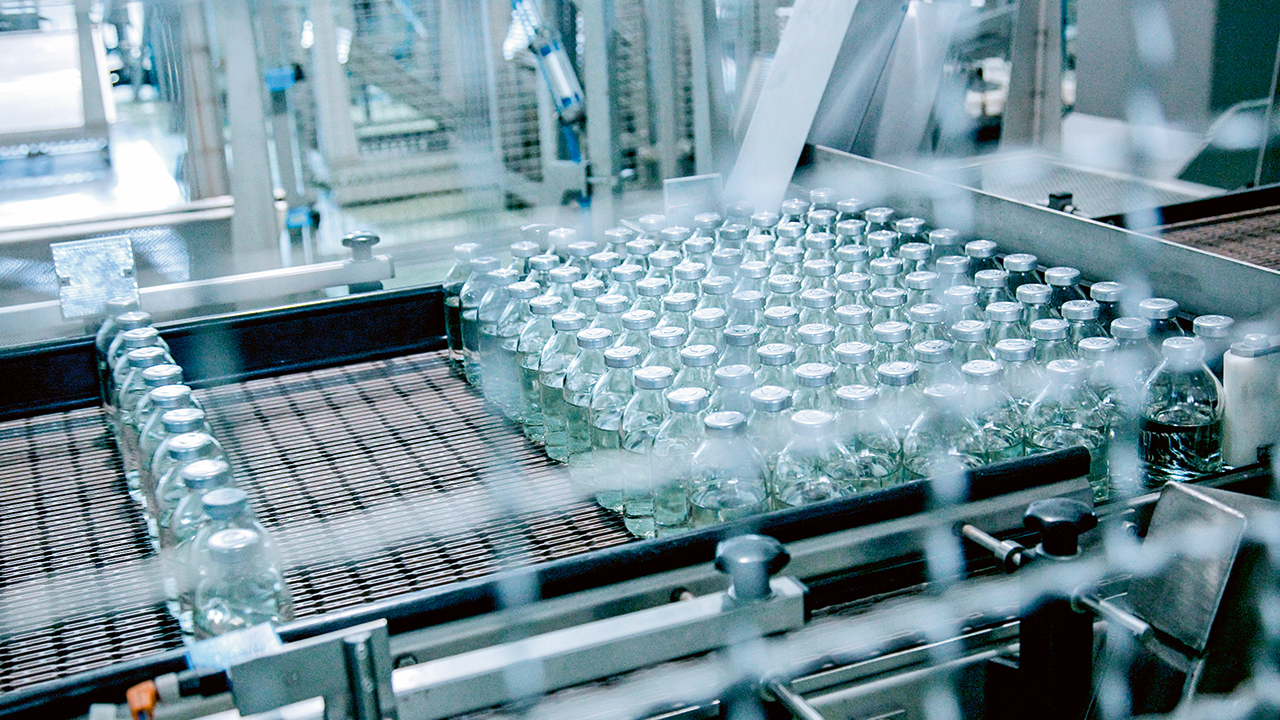
Online batch monitoring
ABB Ability™ BatchInsight’s online alignment algorithms allow batches that deviate onto a bad trajectory to be dynamically corrected and key process variables inside a corridor derived from previous golden batches can be shown →06. In addition, statistical key performance indicators calculated from multivariate analysis can be used to generate alarms. A challenge faced during online monitoring is that it is often unclear how far the process has already progressed.
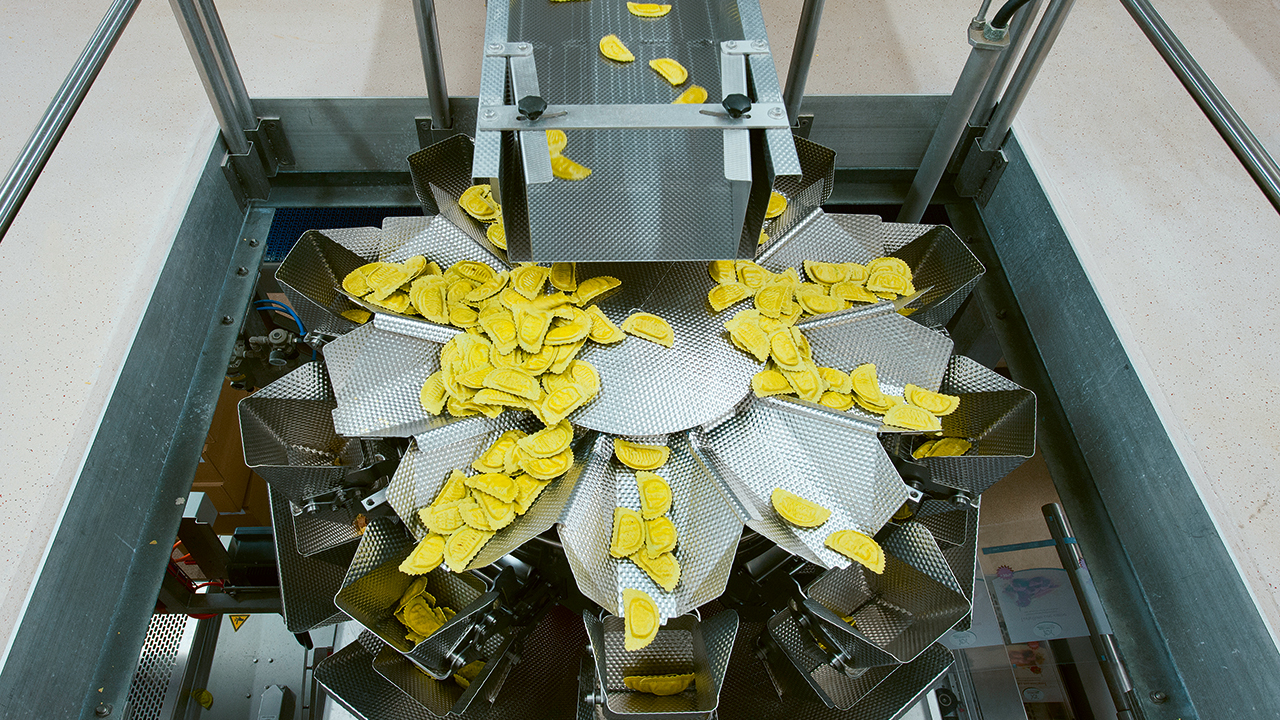
Feedback from the application partner
Tests were run together with the end user (a batch chemical plant) with historical batch data emulating an online approach (meaning data for the current batch was only available until the current step). The MPCA model was used to detect a foaming problem that occurred in a fraction of the batches. The model was trained only with batches without foaming. In 83 percent of the cases, the system was able to predict a foaming event at least 5 minutes before it occurred. Often it predicted the foaming hours before. Although foaming was wrongly predicted in 17 percent of the cases, the predictions are very helpful as the operator can then focus on suspect batches.
Keeping the batch on track
The ABB Ability™ BatchInsight online operator assistant, developed and validated in close collaboration with an application partner, allows the early detection of process abnormalities and the isolation of the process variables explaining them. An operator may be responsible for several batches running in parallel production lines or even have other tasks inside the plant. Here, ABB Ability™ BatchInsight provides a much appreciated tool that alerts operators and enables them to react in a timely fashion to an abnormal situation – here, foaming events – by adjusting the correct process variable. Such timely interventions lead to a reduction of off-specification product, energy and wasted production time.
References
[1] W. Otten, “Industrie 4.0 und Digitalisierung,” atp Magazin, volume 58, number 1 – 2, pp. 28 – 32, 2016.
[2] I. Nimmo, “Operator effectiveness and the human side of error,” CreateSpace Independent Publishing Platform, 2015.
[3] M. Atzmueller et al., “Big data analytics for proactive industrial decision support,” atp Magazin, volume 58, number 9, pp. 62 – 74, 2016.